What are the trends in deep learning for 2023? The simplest answer to this question is: countless. Growing research in deep learning, advancing technologies and increasing computational power make it possible to prototype and put into production systems that even a few years ago were prohibitively expensive. Here are some of the most interesting applications for 2023.
Personal Protective Equipment Detection (Safety)
In smart manufacturing, worker safety is still a very common problem. It is not so much a question of defining the necessary measures, but of monitoring compliance with them. Introducing machine vision within a dense camera network would enable widespread and early monitoring of risk situations such as a helmet not being worn. The possibility of obtaining such information opens up the opportunity to build an effective notification and warning system to prevent accidents at work. The computer vision models used identify people and their PPE, providing information about the correct use of them.
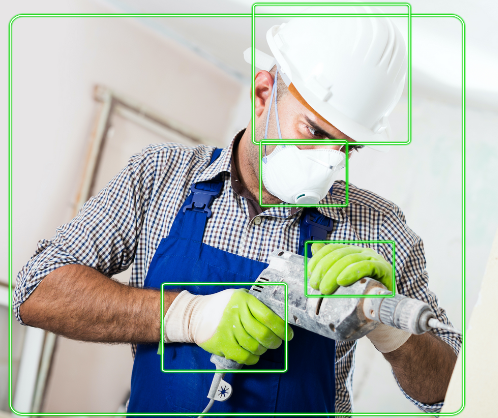
Cancer Detection (Healthcare)

Ref: Artificial Intelligence–Based Breast Cancer Nodal Metastasis Detection: Insights Into the Black Box for Pathologists, Yun Liu, PhD
Deep Learning is rapidly landing in medical industries for many purposes. Some of them include brain, breast and skin cancer detection. One application that is showing promising results is the increasingly accurate classification of tumor cells, which allows them to be distinguished from normal tissues, thus improving early diagnosis and consequently patients’ life expectancy. The models used classify tissues from magnetic resonance images (MRI) with remarkable sensitivity and specificity.
Plant Desease Detection (Agricolture)
The advent of deep learning has also opened up new scenarios in the field of agriculture. The detection of plant diseases is of vital importance for their care, food safety and the prevention of harvest losses. The deep learning methods being developed enable the reduction of costs and time manual inspection of plants by humans, as well as far surpassing the traditional Computer Vision methodologies based on extensive feature engineering and threshold-based image segmentation. An example of a model currently already developed is that of apple black rot recognition.
Customer Attention Tracking (Retail)
Remarkable progress has been made in deep learning with regard to the behavioral analysis of individuals. There are real subject areas focused on: person reidentification, action detection and sentiment detection. In the retail sector, in recent years, much effort has been placed on person reidentification, with the development of models and architectures capable of extracting the appearance features of subjects and thus allowing the reconstruction of the footfall of each individual through videos from multiple cameras installed in a store. Future improvements concern the robustness of reidentification systems, the ability to perform well in crowds, queue analysis and customer purchasing habits.
Infrastructure Condition Monitoring (Transportation)
Infrastructure monitoring makes it possible to identify defects in facilities, prevent accidents, and plan repair activities. A practical example is the monitoring of road surface defects. Typically, this activity requires large personnel costs, sophisticated tools and long lead times: it is carried out using expensive data-collection vehicles or foot-on-ground surveys. A deep learning approach allows the use of computer vision models, also combined with 3D data analysis models, to detect and estimate the extent and severity of road pavement defects.
This article has been written by Giovanni Nardini – R&D Artificial Intelligence Lead